Tune Loggers (tune.logger)#
Tune automatically uses loggers for TensorBoard, CSV, and JSON formats. By default, Tune only logs the returned result dictionaries from the training function.
If you need to log something lower level like model weights or gradients, see Trainable Logging.
Note
Tune’s per-trial Logger
classes have been deprecated. Use the LoggerCallback
interface instead.
LoggerCallback Interface (tune.logger.LoggerCallback)#
Base class for experiment-level logger callbacks |
Handle logging when a trial starts. |
|
Handle logging when a trial restores. |
|
Handle logging when a trial saves a checkpoint. |
|
Handle logging when a trial reports a result. |
|
Handle logging when a trial ends. |
Tune Built-in Loggers#
Logs trial results in json format. |
|
Logs results to progress.csv under the trial directory. |
|
TensorBoardX Logger. |
MLFlow Integration#
Tune also provides a logger for MLflow.
You can install MLflow via pip install mlflow
.
See the tutorial here.
|
MLflow Logger to automatically log Tune results and config to MLflow. |
Wandb Integration#
Tune also provides a logger for Weights & Biases.
You can install Wandb via pip install wandb
.
See the tutorial here.
|
Weights and biases (https://www.wandb.ai/) is a tool for experiment tracking, model optimization, and dataset versioning. |
Comet Integration#
Tune also provides a logger for Comet.
You can install Comet via pip install comet-ml
.
See the tutorial here.
|
CometLoggerCallback for logging Tune results to Comet. |
Aim Integration#
Tune also provides a logger for the Aim experiment tracker.
You can install Aim via pip install aim
.
See the tutorial here
Aim Logger: logs metrics in Aim format. |
Other Integrations#
Viskit#
Tune automatically integrates with Viskit via the CSVLoggerCallback
outputs.
To use VisKit (you may have to install some dependencies), run:
$ git clone https://github.com/rll/rllab.git
$ python rllab/rllab/viskit/frontend.py ~/ray_results/my_experiment
The non-relevant metrics (like timing stats) can be disabled on the left to show only the relevant ones (like accuracy, loss, etc.).
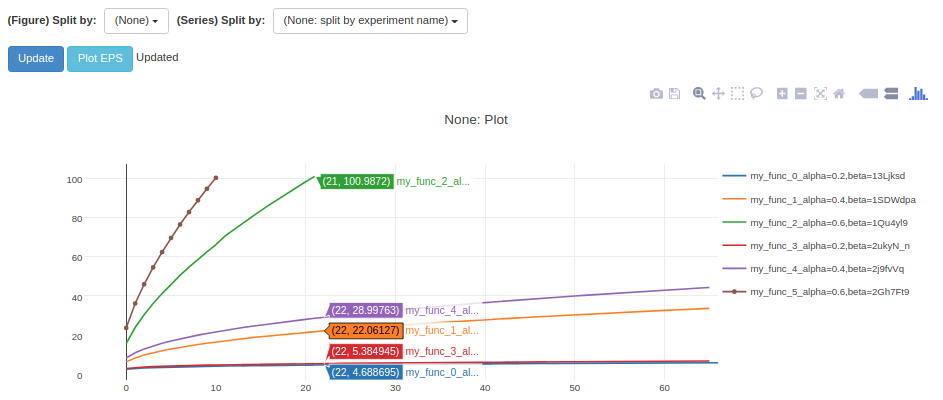