Key Concepts of Ray Tune#
Let’s quickly walk through the key concepts you need to know to use Tune. If you want to see practical tutorials right away, go visit our user guides. In essence, Tune has six crucial components that you need to understand.
First, you define the hyperparameters you want to tune in a search space
and pass them into a trainable
that specifies the objective you want to tune.
Then you select a search algorithm
to effectively optimize your parameters and optionally use a
scheduler
to stop searches early and speed up your experiments.
Together with other configuration, your trainable
, search algorithm, and scheduler are passed into Tuner
,
which runs your experiments and creates trials
.
The Tuner
returns a ResultGrid
to inspect your experiment results.
The following figure shows an overview of these components, which we cover in detail in the next sections.
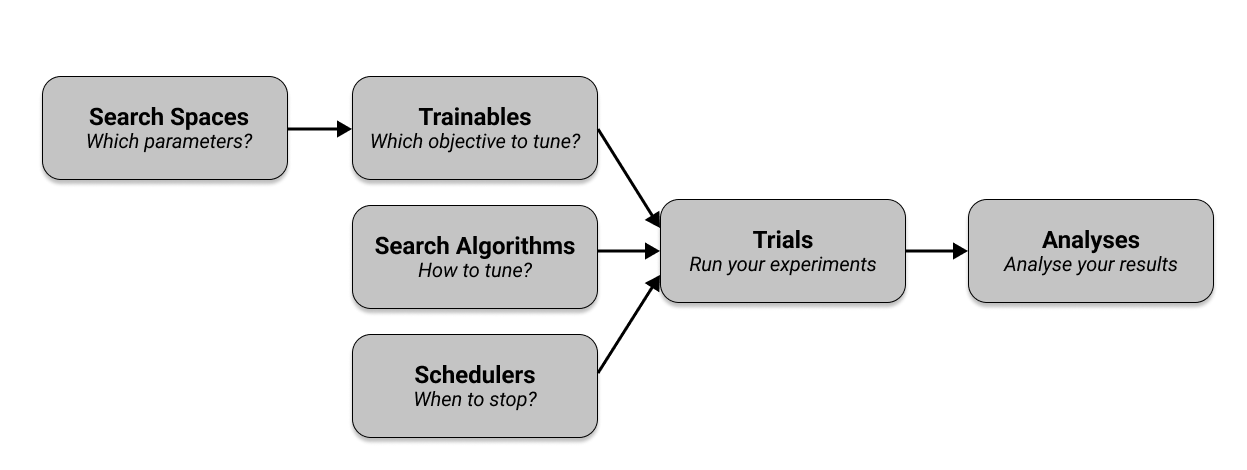
Ray Tune Trainables#
In short, a Trainable is an object that you can pass into a Tune run.
Ray Tune has two ways of defining a trainable
, namely the Function API
and the Class API.
Both are valid ways of defining a trainable
, but the Function API is generally recommended and is used
throughout the rest of this guide.
Consider an example of optimizing a simple objective function like a * (x ** 2) + b `` in which ``a
and b
are the
hyperparameters we want to tune to minimize
the objective.
Since the objective also has a variable x
, we need to test for different values of x
.
Given concrete choices for a
, b
and x
we can evaluate the objective function and get a score
to minimize.
With the the function-based API you create a function (here called trainable
) that
takes in a dictionary of hyperparameters.
This function computes a score
in a “training loop” and reports
this score back to Tune:
from ray import tune
def objective(x, a, b): # Define an objective function.
return a * (x**2) + b
def trainable(config): # Pass a "config" dictionary into your trainable.
for x in range(20): # "Train" for 20 iterations and compute intermediate scores.
score = objective(x, config["a"], config["b"])
tune.report({"score": score}) # Send the score to Tune.
Note that we use tune.report(...)
to report the intermediate score
in the training loop, which can be useful
in many machine learning tasks.
If you just want to report the final score
outside of this loop, you can simply return the score at the
end of the trainable
function with return {"score": score}
.
You can also use yield {"score": score}
instead of tune.report()
.
Here’s an example of specifying the objective function using the class-based API:
from ray import tune
def objective(x, a, b):
return a * (x**2) + b
class Trainable(tune.Trainable):
def setup(self, config):
# config (dict): A dict of hyperparameters
self.x = 0
self.a = config["a"]
self.b = config["b"]
def step(self): # This is called iteratively.
score = objective(self.x, self.a, self.b)
self.x += 1
return {"score": score}
Tip
tune.report
can’t be used within a Trainable
class.
Learn more about the details of Trainables here
and have a look at our examples.
Next, let’s have a closer look at what the config
dictionary is that you pass into your trainables.
Tune Search Spaces#
To optimize your hyperparameters, you have to define a search space. A search space defines valid values for your hyperparameters and can specify how these values are sampled (e.g. from a uniform distribution or a normal distribution).
Tune offers various functions to define search spaces and sampling methods. You can find the documentation of these search space definitions here.
Here’s an example covering all search space functions. Again, here is the full explanation of all these functions.
config = {
"uniform": tune.uniform(-5, -1), # Uniform float between -5 and -1
"quniform": tune.quniform(3.2, 5.4, 0.2), # Round to multiples of 0.2
"loguniform": tune.loguniform(1e-4, 1e-1), # Uniform float in log space
"qloguniform": tune.qloguniform(1e-4, 1e-1, 5e-5), # Round to multiples of 0.00005
"randn": tune.randn(10, 2), # Normal distribution with mean 10 and sd 2
"qrandn": tune.qrandn(10, 2, 0.2), # Round to multiples of 0.2
"randint": tune.randint(-9, 15), # Random integer between -9 and 15
"qrandint": tune.qrandint(-21, 12, 3), # Round to multiples of 3 (includes 12)
"lograndint": tune.lograndint(1, 10), # Random integer in log space
"qlograndint": tune.qlograndint(1, 10, 2), # Round to multiples of 2
"choice": tune.choice(["a", "b", "c"]), # Choose one of these options uniformly
"func": tune.sample_from(
lambda spec: spec.config.uniform * 0.01
), # Depends on other value
"grid": tune.grid_search([32, 64, 128]), # Search over all these values
}
Tune Trials#
You use Tuner.fit to execute and manage hyperparameter tuning and generate your trials
.
At a minimum, your Tuner
call takes in a trainable as first argument, and a param_space
dictionary
to define the search space.
The Tuner.fit()
function also provides many features such as logging,
checkpointing, and early stopping.
In the example, minimizing a (x ** 2) + b
, a simple Tune run with a simplistic search space for a
and b
looks like this:
# Pass in a Trainable class or function, along with a search space "config".
tuner = tune.Tuner(trainable, param_space={"a": 2, "b": 4})
tuner.fit()
Tuner.fit
will generate a couple of hyperparameter configurations from its arguments,
wrapping them into Trial objects.
Trials contain a lot of information.
For instance, you can get the hyperparameter configuration using (trial.config
), the trial ID (trial.trial_id
),
the trial’s resource specification (resources_per_trial
or trial.placement_group_factory
) and many other values.
By default Tuner.fit
will execute until all trials stop or error.
Here’s an example output of a trial run:
== Status ==
Memory usage on this node: 11.4/16.0 GiB
Using FIFO scheduling algorithm.
Resources requested: 1/12 CPUs, 0/0 GPUs, 0.0/3.17 GiB heap, 0.0/1.07 GiB objects
Result logdir: /Users/foo/ray_results/myexp
Number of trials: 1 (1 RUNNING)
+----------------------+----------+---------------------+-----------+--------+--------+----------------+-------+
| Trial name | status | loc | a | b | score | total time (s) | iter |
|----------------------+----------+---------------------+-----------+--------+--------+----------------+-------|
| Trainable_a826033a | RUNNING | 10.234.98.164:31115 | 0.303706 | 0.0761 | 0.1289 | 7.54952 | 15 |
+----------------------+----------+---------------------+-----------+--------+--------+----------------+-------+
You can also easily run just 10 trials by specifying the number of samples (num_samples
).
Tune automatically determines how many trials will run in parallel.
Note that instead of the number of samples, you can also specify a time budget in seconds through time_budget_s
,
if you set num_samples=-1
.
tuner = tune.Tuner(
trainable, param_space={"a": 2, "b": 4}, tune_config=tune.TuneConfig(num_samples=10)
)
tuner.fit()
Finally, you can use more interesting search spaces to optimize your hyperparameters
via Tune’s search space API, like using random samples or grid search.
Here’s an example of uniformly sampling between [0, 1]
for a
and b
:
space = {"a": tune.uniform(0, 1), "b": tune.uniform(0, 1)}
tuner = tune.Tuner(
trainable, param_space=space, tune_config=tune.TuneConfig(num_samples=10)
)
tuner.fit()
To learn more about the various ways of configuring your Tune runs, check out the Tuner API reference.
Tune Search Algorithms#
To optimize the hyperparameters of your training process, you use a Search Algorithm which suggests hyperparameter configurations. If you don’t specify a search algorithm, Tune will use random search by default, which can provide you with a good starting point for your hyperparameter optimization.
For instance, to use Tune with simple Bayesian optimization through the bayesian-optimization
package
(make sure to first run pip install bayesian-optimization
), we can define an algo
using BayesOptSearch
.
Simply pass in a search_alg
argument to tune.TuneConfig
, which is taken in by Tuner
:
from ray.tune.search.bayesopt import BayesOptSearch
# Define the search space
search_space = {"a": tune.uniform(0, 1), "b": tune.uniform(0, 20)}
algo = BayesOptSearch(random_search_steps=4)
tuner = tune.Tuner(
trainable,
tune_config=tune.TuneConfig(
metric="score",
mode="min",
search_alg=algo,
),
run_config=tune.RunConfig(stop={"training_iteration": 20}),
param_space=search_space,
)
tuner.fit()
Tune has Search Algorithms that integrate with many popular optimization libraries, such as HyperOpt or Optuna. Tune automatically converts the provided search space into the search spaces the search algorithms and underlying libraries expect. See the Search Algorithm API documentation for more details.
Here’s an overview of all available search algorithms in Tune:
SearchAlgorithm |
Summary |
Website |
Code Example |
---|---|---|---|
Random search/grid search |
|||
Bayesian/Bandit Optimization |
[Ax] |
||
Tree-Parzen Estimators |
[HyperOpt] |
||
Bayesian Optimization |
|||
Bayesian Opt/HyperBand |
[BOHB] |
||
Gradient-free Optimization |
|||
Optuna search algorithms |
[Optuna] |
Note
Unlike Tune’s Trial Schedulers, Tune Search Algorithms cannot affect or stop training processes. However, you can use them together to early stop the evaluation of bad trials.
In case you want to implement your own search algorithm, the interface is easy to implement, you can read the instructions here.
Tune also provides helpful utilities to use with Search Algorithms:
Repeated Evaluations (tune.search.Repeater): Support for running each sampled hyperparameter with multiple random seeds.
ConcurrencyLimiter (tune.search.ConcurrencyLimiter): Limits the amount of concurrent trials when running optimization.
Shim Instantiation (tune.create_searcher): Allows creation of the search algorithm object given a string.
Note that in the example above we tell Tune to stop
after 20
training iterations.
This way of stopping trials with explicit rules is useful, but in many cases we can do even better with
schedulers
.
Tune Schedulers#
To make your training process more efficient, you can use a Trial Scheduler.
For instance, in our trainable
example minimizing a function in a training loop, we used tune.report()
.
This reported incremental
results, given a hyperparameter configuration selected by a search algorithm.
Based on these reported results, a Tune scheduler can decide whether to stop the trial early or not.
If you don’t specify a scheduler, Tune will use a first-in-first-out (FIFO) scheduler by default, which simply
passes through the trials selected by your search algorithm in the order they were picked and does not perform any early stopping.
In short, schedulers can stop, pause, or tweak the hyperparameters of running trials, potentially making your hyperparameter tuning process much faster. Unlike search algorithms, Trial Scheduler do not select which hyperparameter configurations to evaluate.
Here’s a quick example of using the so-called HyperBand
scheduler to tune an experiment.
All schedulers take in a metric
, which is the value reported by your trainable.
The metric
is then maximized or minimized according to the mode
you provide.
To use a scheduler, just pass in a scheduler
argument to tune.TuneConfig
, which is taken in by Tuner
:
from ray.tune.schedulers import HyperBandScheduler
# Create HyperBand scheduler and minimize the score
hyperband = HyperBandScheduler(metric="score", mode="max")
config = {"a": tune.uniform(0, 1), "b": tune.uniform(0, 1)}
tuner = tune.Tuner(
trainable,
tune_config=tune.TuneConfig(
num_samples=20,
scheduler=hyperband,
),
param_space=config,
)
tuner.fit()
Tune includes distributed implementations of early stopping algorithms such as Median Stopping Rule, HyperBand, and ASHA. Tune also includes a distributed implementation of Population Based Training (PBT) and Population Based Bandits (PB2).
Tip
The easiest scheduler to start with is the ASHAScheduler
which will aggressively terminate low-performing trials.
When using schedulers, you may face compatibility issues, as shown in the below compatibility matrix. Certain schedulers cannot be used with search algorithms, and certain schedulers require that you implement checkpointing.
Schedulers can dynamically change trial resource requirements during tuning. This is implemented in ResourceChangingScheduler, which can wrap around any other scheduler.
Scheduler |
Need Checkpointing? |
SearchAlg Compatible? |
Example |
---|---|---|---|
No |
Yes |
||
No |
Yes |
||
Yes |
Yes |
||
Yes |
Only TuneBOHB |
||
Yes |
Not Compatible |
||
Yes |
Not Compatible |
Learn more about trial schedulers in the scheduler API documentation.
Tune ResultGrid#
Tuner.fit()
returns an ResultGrid object which has methods you can use for
analyzing your training.
The following example shows you how to access various metrics from an ResultGrid
object, like the best available
trial, or the best hyperparameter configuration for that trial:
tuner = tune.Tuner(
trainable,
tune_config=tune.TuneConfig(
metric="score",
mode="min",
search_alg=BayesOptSearch(random_search_steps=4),
),
run_config=tune.RunConfig(
stop={"training_iteration": 20},
),
param_space=config,
)
results = tuner.fit()
best_result = results.get_best_result() # Get best result object
best_config = best_result.config # Get best trial's hyperparameters
best_logdir = best_result.path # Get best trial's result directory
best_checkpoint = best_result.checkpoint # Get best trial's best checkpoint
best_metrics = best_result.metrics # Get best trial's last results
best_result_df = best_result.metrics_dataframe # Get best result as pandas dataframe
This object can also retrieve all training runs as dataframes, allowing you to do ad-hoc data analysis over your results.
# Get a dataframe with the last results for each trial
df_results = results.get_dataframe()
# Get a dataframe of results for a specific score or mode
df = results.get_dataframe(filter_metric="score", filter_mode="max")
See the result analysis user guide for more usage examples.
What’s Next?#
Now that you have a working understanding of Tune, check out:
User Guides: Tutorials for using Tune with your preferred machine learning library.
Ray Tune Examples: End-to-end examples and templates for using Tune with your preferred machine learning library.
Getting Started with Ray Tune: A simple tutorial that walks you through the process of setting up a Tune experiment.
Further Questions or Issues?#
You can post questions or issues or feedback through the following channels:
Discussion Board: For questions about Ray usage or feature requests.
GitHub Issues: For bug reports.
Ray Slack: For getting in touch with Ray maintainers.
StackOverflow: Use the [ray] tag questions about Ray.